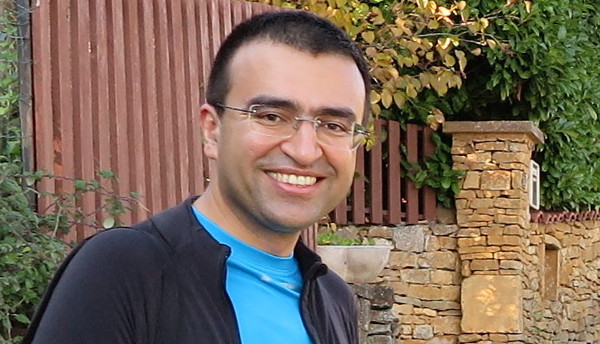
ABSTRACT: Astronomical instrumentation has greatly advanced over the last 40 years: for example with digital detectors, space telescopes and +8m class ground-based telescopes. However, the signal-based detection paradigm (for example from Petrosian or Kron in the 1970s, mostly used as implemented in SExtractor from the mid-1990s) is still the dominant method of low-level data analysis: detection, segmentation and measurements (or catalog production). In this talk, after reviewing the major systematic biases regarding astronomical object detection that is inherent to the signal-based paradigm, I will introduce a fundamentally different noise-based detection paradigm for detecting signal that may have extremely low signal-to-noise ratios with any shapes (e.g., galaxies!). With thresholds that are far below the Sky value, and non-parametric expansion into noise, it is successfully able to detect very diffuse and irregularly shaped signal in noise. As a result, it has been shown to find the sky level to almost one order of magnitude higher precision. The software implementation is called NoiseChisel (which is distributed as part of GNU Astronomy Utilities; or Gnuastro; see links below). The potentials for testing and using it in wide-field surveys like the China Station Space Telescope (CSST) will be discussed; in particular based on lessons learnt after being included in the pipeline of the European Space Agency's Euclid mission and usage in several other ground-based data reduction and analysis pipelines.
Relevant links:
NoiseChisel paper I: https://arxiv.org/abs/1505.01664
NoiseChisel paper II: https://arxiv.org/abs/1909.11230
Gnuastro main webpage: https://www.gnu.org/software/gnuastro
Available in Conda: https://anaconda.org/conda-forge/gnuastro
Video Tutorial: https://peertube.stream/w/cdf2467c-bf6c-4462-93f4-9900067913cf
NoiseChisel handson: https://peertube.stream/w/35b7c398-9fd7-4bcf-8911-1e01c5124585
Gnuastro docs (HTML): https://www.gnu.org/s/gnuastro/manual/html_node
Gnuastro docs (PDF): https://www.gnu.org/s/gnuastro/manual/gnuastro.pdf
Tutorial: https://www.gnu.org/software/gnuastro/manual/html_node/General-program-usage-tutorial.html